Can we change perception in pitch darkness? Can we bring that daytime type of situational awareness to the nighttime? Based on recent breakthroughs in processing thermal imaging using artificial intelligence and machine learning, a team of researchers from Purdue University and Michigan State University believe the answer is yes. The group recently published the results of their recent work in Nature, in which they believe they have solved the “ghosting” problem present in thermal imaging.
Using AI and ML algorithms to process thermal imaging scans, the group believes this method, heat-assisted detection and ranging, or HADAR, could revolutionize the autonomous vehicle and robotics spaces, as well as impact geosciences.
That is according to Zubin Jacob, the Elmore Associate Professor of Electrical and Computer Engineering in the Elmore Family School of Electrical and Computer Engineering at Purdue, one of the authors of the paper. Jacob recently took some time to speak with Geo Week News about the group’s work and HADAR in general, both where things are today and what he sees in the future.
What is ghosting?
Before getting into the algorithm researchers devised and the resulting image, what they’re calling TeX-Net and TeX-Vision, respectively, it’s worth quickly delving into this key problem of “ghosting,” which is the issue of thermal images losing texture. (Point clouds from lidar data also suffer from ghosting issues, albeit for different reasons and generally to a lesser extent.) Jacob describes the issue using the example of a light bulb.
“If you have a light bulb with something written on top of it, or there's some texture on top of it, if the light bulb is on, then you cannot read it because there's lots of light coming from the bulb itself,” Jacob said. “But if you switch off the bulb, then what you're seeing is the radiation reflected on the light bulb and reaching your eye.”
That issue of removing the extrinsic radiation – i.e. the radiation coming from the environment that is interacting with an object or material – is crucial so that resulting images only show textures from the objects in question. This kind of accuracy is, of course, crucial for autonomous navigation applications. “In the real world,” Jacob said, “we can never turn off the radiation coming from objects. So we needed some clever way to separate the intrinsic radiation from all the other radiation that is falling on the object and reaching your camera.”
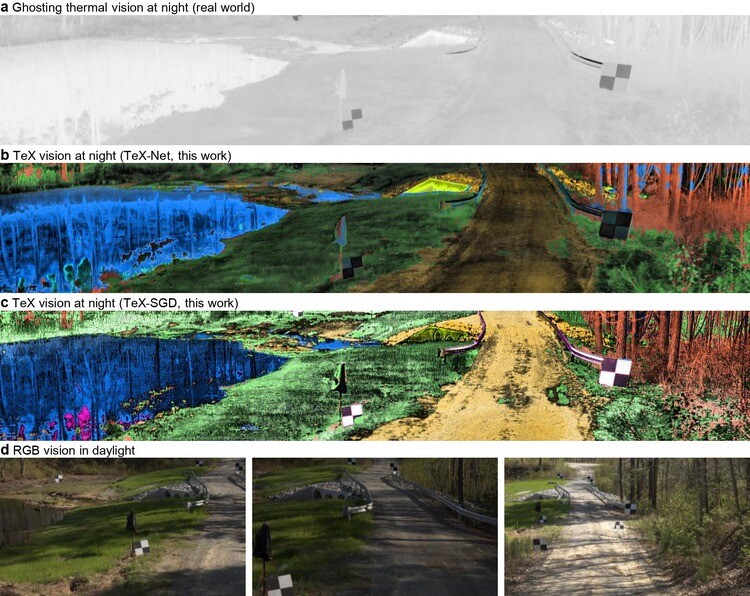
Introducing TeX-Net and TeX-Vision
Jacob and the rest of the team did indeed come up with that clever way with the algorithm they’re calling TeX-Net. The “TeX” part of this stands for temperature, emissivity, and texture, the attributes needed to perceive these scenes. Explaining the machine learning technique, he said, “It is taking in information about the surroundings, and it’s just collecting the thermal images. But it collects the thermal images at maybe 100 different spectral bands, so it’s really 100 thermal images.”
From there, they use a database to estimate the material emissivities, and are currently developing their own database for this. “Then we try to estimate what are the different materials, and try to exploit the spectral emission and the material database to separate the intrinsic and extrinsic radiation. TeX-Net has this machine vision algorithm with thermal physics embedded in it that separates out the intrinsic and extrinsic radiation. Once we do that, we actually get these rather high-quality images, even at night.”
The end result is an image they are calling TeX-Vision. TeX-Vision, he says, “is actually a representation of the thermal radiation data that is coming at you without emitting any sensor signals. It’s a completely passive approach.” Jacob also notes that while traditional cameras generally work on three frequencies – red, blue, and green – since the infrared spectral range is invisible to the eye “there’s no reason to pick three frequencies. We are losing information if we just choose three frequencies, so the idea is to collect as many bands as possible.”
And then they need to represent those findings. Jacob says the team was inspired by point clouds as a representation of lidar data, and found a way to visualize those three aforementioned properties: temperature, emissivity, and texture. They map the data to the HSV format (hue, saturation, and value), resulting in accurate and colorful images of a scene.
“When we look at it, it’s very interesting,” Jacob tells Geo Week News, “because grass will always look like grass. When the temperature changes the grass may have different texture, but the color representation is always going to be green because the emissivity is fixed. “e” fixes the color. Only the hue changes when the temperature changes, so this representation is extremely useful.”
What’s next?
To this point, the work from Jacob and the other researchers has been in the proof-of-concept and experimental realm, and there are still a few barriers that need to be overcome before it can be a practical real-world tool. First and foremost is the size of the scanners. Right now, Jacob says the scanners used for HADAR are about the size of a microwave oven, with the goal being to bring it down to something that can fit in the palm of someone’s hand. That can’t happen until they figure a way to remove the need for cooling in these machines.
Additionally, and connecting back to the size issue, Jacob notes that they need to enable real-time processing, particularly for the autonomous vehicle and robotics applications. Right now, they are “roughly in the ballpark of a couple of minutes,” with variance based on the complexity of a scene and whether there is a known ground truth. This again comes down to size, as they need to be able to fit computing within the same unit to make it work.
Those kinds of issues are to be expected with work so new and experimental, but it does have the potential to change the way we perceive environments in the dark, even compared to lidar and especially when compared to traditional cameras. Asked if he envisions this as a standalone tool or used in concert with other sensors, Jacob said, “I have a feeling that the best option is using it in conjunction with low-cost, sparse lidar. We have this TeX-Vision where we have a good three-dimensional understanding of the scene, but it’s not perfect. For some points, we use lidar and we are really able to really reconstruct the scene to what a high-resolution lidar would do, but then we have the additional advantage that there’s a lot of semantic information such as temperature, emissivity, and others.”
Broadly, what does Jacob see as the future for HADAR? “I’d say that this is a way of going to the next generation of thermal imaging.”