“Why do we talk about 3D as being equivalent to a point cloud or to a triangle mesh?” Radu Rusu, CEO and co-founder of Fyusion asks me over Skype.
Rusu is uniquely positioned to pose—and answer—that question. He cut his teeth at the famed Willow Garage robotics laboratory where he helped develop crucial 3D industry tools such as Open CV and PCL, the latter being an open-source library of point cloud algorithms used very widely for 3D computer vision processing.
Point clouds and triangle meshes, he tells me, are excellent data formats for many professional uses, but inadequate for consumers, who require more realism. Photos and videos are better for consumers, but all of the above are less than ideal for training machine-learning algorithms or visualizing objects in AR and VR. “So we thought we would use a completely new paradigm, a clean slate,” says Rusu. “We’re experts in point cloud processing, and we’ve done all the work in computer vision. Can we put it together and make something a little different that would satisfy the criteria we require these days?”
The result was a new 3D format named Fyuse. Rusu says that this format, which he describes as being like a “3D JPEG,” will enable any camera to capture more than just 3D content, but “3D spatial photography,” and to glean a rich 3D understanding of the world in real time. We spent the rest of our conversation unpacking just what that meant.
Before you dig in, play around with the Fyuse embedded below for some context.
FYU.add(“cpx93pws0nws”, “fyu1”, {preload:1,autoplay:0,zoom:1,zoomBtns:1,inlineBtn:1});
Fyuse
When you use Fyuse for the first time, the capture process will probably look something like this: You move your smartphone around the object, and the system processes the images alongside data from the other sensors in your phone, like the accelerometer, to create a 3D model.
The approach has a superficial similarity to photogrammetry, Rusu admits, but it offers results much richer than textured meshes. Fyuse also logs information like camera position, inertial measurement unit data, and optical flow, and stores them all in the same Fyuse data container. All this extra information allows Fyuse to “decouple” the rendering and the visual aspects. When a user opens a Fyuse file and “looks” at the object from a specific angle, a “mini rendering engine under the hood” reads the container to get the data required for the user’s POV, and then renders it. The result is a remarkably realistic, infinitely smooth model–with the added benefit of 3D point cloud data underneath for measurement and other needs.
Rusu compares the operation of the Fyuse platform to light field capture, or what he calls “3D v2.0.” (Light field technology, a primary focus of Google’s recent VR efforts, uses an array of cameras to capture all the rays of light moving through a particular volume of space, and then fuses them into a single file. Light field field files are great to view in VR because a user with a headset on can move her head within that space freely, and from each vantage get a photorealistic view.)
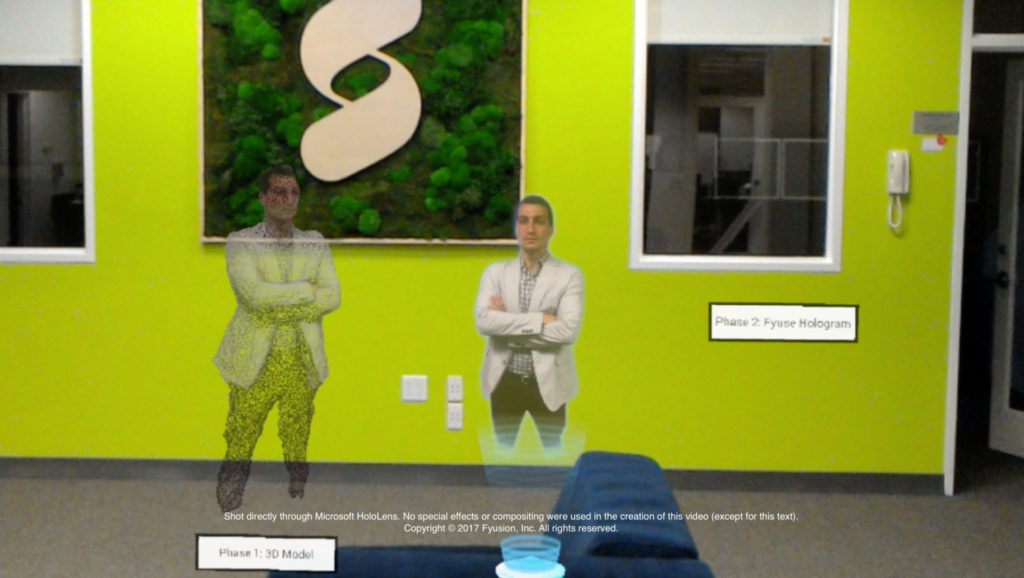
Left: A triangle mesh as viewed through HoloLens. Right: A Fyuse rendering as viewed through HoloLens.
Better visualization
This light field approach makes files that simply look better than a textured mesh, which Rusu calls “3D v1.0.” He explains that triangle meshes with a single texture draped over them are not capable of a photorealistic look because they blend textures in a way that can’t achieve high fidelity. “It goes into the uncanny valley,” Rusu says. “I’ve never seen a human being who looks right, even when captured with an array of SLRs.” Furthermore, he argues, objects that can’t be modeled in 3D—mirrors, glass, anything translucent, objects that are far away, water, the sky—can’t be put in the mesh.
3D visual understanding, better machine learning
This is only half the story. “Fyuse is a format that we built specifically for machine learning,” Rusu says, “forward-facing and compatible with new hardware devices.” As such, it has enabled the company to design versatile, high-performance machine learning algorithms so fast that they can operate in the capture device in real time. He demonstrates by showing me a video of the software projecting a skeleton onto a live video of a person dancing.
The new Fyuse format makes this kind of speed possible by offering better data for training the machine-learning algorithms. Historically, such algorithms have been trained with uncorrelated 2D data, meaning that each image is treated as a distinct frame that is unrelated to every other frame. This requires you to feed each algorithm a huge amount of data about, say, shoes, before it can build a good model for recognizing shoes. Though object-recognition models trained in this manner get very good, they also get very big, and then require a lot of computing resources to run. The Fyuse format offers rich, spatially correlated data, which allows machine-learning algorithms to build accurate object-recognition models while remaining lean enough to operate in real time on a mobile device with very limited computing power.
It is a tech cliché in 2018, but in this case it’s true: The Fyuse format enables the use of machine-learning algorithms that operate more like humans do. “Think about what happens in our brains,” Rusu says. “We don’t process individual ‘optical frames’ in isolation and then discard the results from the previous frame when we process the current one. Instead, we continuously refine our interpretation of visual data in an iterative process, and improve our cognitive model representations over time.”
“By seeing objects from multiple angles and building their geometric models over time,” he continues, “together with redefining machine learning to work on light field data rather than 2D, we’re able to build much better machine learning models, with better performance and robustness, that can be run live in the camera on mobile devices.”
This feature is “baked in” to the Fyuse format, and gives all smartphones and AR headsets the ability to have a “3D visual understanding” of their environments in real time.
Next steps
Fyusion knows that the transition from great idea to viable product has to be undertaken slowly and carefully.
The company started by building up a consumer app to prove the viability of their new format. When they saw the user base grow beyond expectations–reaching a peak of more than 30 million–they began building out the Fyusion platform for enterprise verticals. This includes e-commerce and automotive products offer quick, high-quality product visualization, and the opportunity to perform functions like putting your car in a different environment. It also includes AR products that allow users to put those fast machine-learning algorithms to work to put costumes on themselves, give themselves wings, or alter their appearance in any number of ways. Fyusion is also working to get the new format “baked in” to your next smartphone, where it could become just as ubiquitous as the jpegs you shoot with your digital camera.
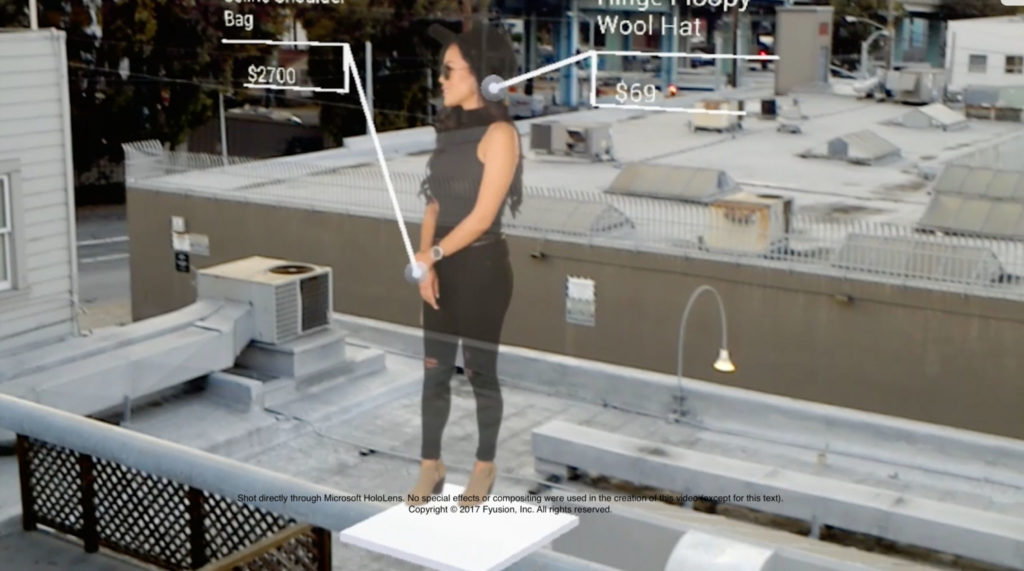
Fyuse used in an AR e-commerce application.
However, Rusu tells me that the Fyuse file format should be useful far beyond this limited list of applications. He says the algorithms his team has already built can be trained quickly to work for a potentially massive number of applications, like gathering a quick 3D understanding of people, cars, trees, scenes, and really anything you want. “The algorithms don’t change at all,” he says, “nothing else changes but the data.”
In closing Rusu reminds me that the company’s long-term vision was to “pioneer 3D real time visual understanding of the physical world around us, using any camera, and to build 3D spatial photography for consumers at scale.” Instead of seeing “point clouds and 3D meshes as the solutions to all problems,” they have moved on to a new paradigm for 3D, and opened up a whole raft of potential applications.
For more information, or to discuss the possibilities of the format, see Fyusion.com
FYU.add(“btnv2am5z1”, “fyu_btnv2am5z1”);