3D data capture is being employed as a matter of course in the public safety arena. By documenting accident scenes and forensic laser scanning to understand how accidents and intentional bad acts occur, for example, 3D information can deliver invaluable information to law enforcement and first responders.
The idea is simple: If we can know how bad things happened, we can work to prevent them from happening again.
But what about using 3D data in real time? Can we capture 3D data in such as way as to deliver information as an event is happening so that first responders can more effectively save lives and prevent tragedy?
Almost certainly. But there’s some question still about the best way to do it.
Testing the use cases for 3D mapping in disaster and first response
Leading the charge in the United States to figure this out is the National Institute of Standards and Technology (NIST). This effort includes NIST’s Public Safety Communications Research Division, as well as the Global City Teams Challenge (GCTC) that NIST organizes in conjunction with the U.S. Department of Homeland Security (DHS) Science and Technology Directorate. Launched in 2014, the GCTC is an effort to encourage development of standards-based smart city applications that provide “measurable benefits” to society at large.
By 2018-2019, DHS created a new focus on a Smart and Secure Cities and Communities Challenge (SC3), which encouraged cybersecurity and privacy to be top of mind during any application.
There are now some 200 “action clusters” participating in the program, which generally involve a city’s government and any number of companies and academic institutions. They’re working to solve all manner of problems, from quick and efficient mass notification systems to reduction of light pollution to addressing the climate crisis.
Not surprisingly, more than one of these clusters is focusing on 3D mapping tech and how it might be used to assist first responders.
Point Cloud City Initiative
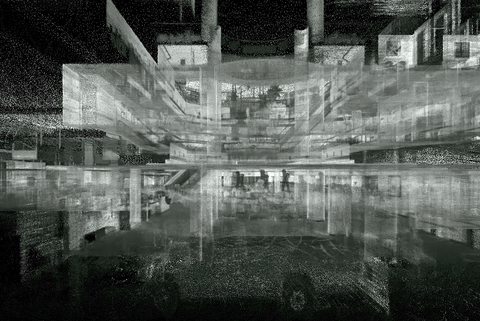
An example indoor point cloud (NIST).
For example, in Memphis, Tenn., there’s a project titled, “Map901: Building Rich Interior Hazard Maps for First Responders,” funded through the NIST Point Cloud City initiative as part of GCTC. It’s pretty much what the title says: Working with the city, Lan Wang, the chair of the computer science department at the University of Memphis has captured the interiors of seven major buildings in Memphis, a total of 1.8 million square feet, with the intention of creating an ArcGIS-based application for first responders to use, which would draw on a catalog of 3D indoor maps.
Chosen for their variety of interior spaces and scanning challenges, the buildings run the gamut from the Memphis Central Library to the Liberty Bowl, and all represent places where large crowds may gather and to which rescue services might have to respond with many lives on the line.
To capture the data, the University of Memphis team used a GVI LiBackpack with Velodyne PUCK lidar, plus an Insta360 Pro 2 camera, along with some temperature, humidity, and sound sensors. Just collecting and displaying the data wasn’t enough — they’re also using neural networks to do automatic image recognition so as to annotate the data with objects of interest for first responders.
The team is using LAS 1.4-R13 format for the point cloud data, allowing for integration of color and user-defined classes, “so we can label the different types of objects in the point cloud,” Wang told a NIST stakeholder meeting this past summer. For the video, they captured in 4K resolution at 30 frames per second in H.264 format.
To put it simply, the data capture alone is “not straightforward,” Wang said. “Most equipment is designed for outdoor mapping. We have to spend a lot of time fine-tuning the devices and learning what’s the best practice for scanning indoor spaces.” The asked anyone in a room to stay still when they came through, and reported great difficulty with small spaces and rooms that had mirrors or reflective surfaces.
For the object recognition, they used Mask R-CNN to create bounding boxes for each object and then the Inception ResNet-v2 algorithm for object labeling, trained to identify safety-related objects like fire extinguishers, exits, windows, and the like.
Joel Lawhead, at N-Vision Solutions, is doing something similar with the Hancock County (MS) Emergency Management Agency, as another grantee in the Point Cloud City project. For their project, they focused on scanning public schools, 30 in all, for a total of 1.2 million square feet of indoor space.
He reiterated the complex nature of trying to create annotated 3D maps for first responder use. “It’s a huge thing,” he said at the NIST stakeholder meeting. “Imagine taking a sharpie and labeling every single thing in this room; and then for every room in the hotel; and then for nine other hotels. Even if you’re using machine learning, you have to train the computer, which is like training a two-year-old.”
The Hancock County project used YOLO for image recognition, but Lawhead noted that it’s much easier to recognize object in imagery than point clouds, “video and image recognition is much farther ahead,” so they did the recognition in the image data, “and then we can layer that onto the 3D data with a mask.”
They used a Zeb-Revo RT scanner, a hand-held device built for indoor mapping, with real-time visibility of the point cloud data.
In the end, said Gertrude Moeller, the project manager for the city of Memphis working with Wang’s team, the city hopes to increase the safety of first responders by having 3D interior maps that can assist in training — even in live situations. “We hope ultimately,” she said, “that we could have a virtual display that would allow firefighters to navigate through a building, because very often the interior is obscured by smoke and that really increases the risk of injury or loss of life for firefighters.”
Ideas on how that would work involve everything from a central command center getting pings on a digital twin of a building when first responders mark an area to something as involved as a heads-up display with augmented reality worn as part of a helmet.
What is best way to integrate all of this 3D data into actual first responder work?
Of course, there’s some question as to whether first responders in the building actually want that kind of heads-up display. Or should the information only go to a centrally located situation manager, who could use the 3D data to guide first responders in the field, collect data from them, and update a situation awareness software of some kind?
That’s what Brenda Bannan, a learning scientist who has recently helped create the Center for Advancing Human-Machine Partnership, is studying at George Mason University. In partnership with the Center for Innovative Technology and Smart City Works in Virginia, Bannan led a live simulation active shooter exercise in November of 2019 with six separate first-responder teams comprising law enforcement, fire, and emergency medical services at GMU’s 10,000-seat EagleBank Arena, which had been scanned for creation of a digital twin.
Could the 3D data, when integrated with all manner of video cameras and sensor data, help the first responders complete tasks faster and improve their response times?
There were clear advantages for incident command. As the individual responders wore location sensors, those who didn’t go into the building were able to see how this data could help organize responders and note exactly where they were in the digital twin. Plus, said Bannan, “you can fly through and see assets of the building and there were occupancy sensors installed so we could see, as the event took place, how many people were there in each area of the building.” Ecodomus, the technology company who provided this capability, permitted interactive viewing between and among sensor data and the digital twin representation to allow the user to navigate through the space while also seeing live video feeds at particular points in the building.
“That’s useful for incident command,” Bannan said, “knowing where the most victims are or seeing that the first responders are clustered together when they may need to spread out in order to treat more patients quickly.”
And that incident command is housing leadership from different responder teams, “and they’re communicating with each other,” said Bannan, “so having them all have a common mental model of what’s happening in the event is really important.”
It’s easy to see which elevator should be shut down, or which doors should be remotely locked to contain a shooter, given a good 3D model of the facility. Or those in the building can quickly be given voice commands to help them get to a location where their help is needed. It was much easier, said Bannan, for first responders to begin to see how to orient themselves vs. a paper map.
On one of the runs, she said, “we had some victims hiding, and those viewing the scene with the digital technology were able to find these victims or patients in a few minutes, very quickly, and the first responders took a lot longer without those tools.”
Further, there is great forensic value for the type of work Bannan does, as she can now use all of the video and analytics gathered from the event to study how groups worked together, how long various tasks took, and other information to help design effective training and give these teams insight on how to be more efficient, improve response times and care for more patients quickly.
For example, “once teams go in,” Bannan said, “they all tend to cluster around those first patients, so if we can spatially show back to them how to better use their resources to locate and divide up amongst all the patients,” that would have clear advantages.
But: “We’re not quite there yet.”
It’s a lot of data that needs to be integrated and they haven’t created the killer app yet that brings it all together and makes it easily accessible.
That’s why she makes a great team with David Lattanzi, a structural engineer and her co-founder of the Center for Innovation and Technology at GMU. He’s doing research into the 3D data capture portion, mostly in the photogrammetry realm, and he was also at the active-shooter drill as an observer.
He noted the digital twin was CAD-based, and wasn’t being updated in real time with the sensor data being collected. It was basically a well-equipped map. Still, the drill was, he said, “one of the most impressive research events I’ve seen in a long time.”
Integrating the 3D data, all of the sensor data, is truly a tough nut to crack.
“You’re talking about making decisions and having them be resolved in less than a minute,” Lattanzi said. “Things happen in that space at light speed. It’s really challenging: How do you give them the data, and who do you give the data to in a way that’s fast enough and useful? I don’t think anyone has the answer to that yet.”
While the heads up displays that Moeller in Memphis is talking about sound like a great idea, no one’s been able to deliver the solution that first responders rave about yet. “What they find when they do this,” Lattanzi said, “is that they lose situational awareness and you’re not able to focus on what you need to focus on. There’s too much information being spit at you.
“It’s very hard to use augmented reality when your life depends on it.”
That’s why the central command was getting the data and relaying it as necessary. But maybe that’s where Bannan and her research can help. What if first responders trained first in a virtual reality environment, using the digital twin of the building to build what’s essentially an incredibly realistic video game, so that they could become used to the information stream? What if they then transitioned to training in an augmented reality environment in the actual building?
And then there is the photogrammetry questions Lattanzi is exploring. Could the digital twin be updated in real time using crowd-sourced 3D data from people inside the building? Could a digital twin essentially be created in real time, again using crowd-sourced information? Could you use devices to add a photorealistic visualization of what’s going on to a pre-made digital twin? The possibilities are seemingly endless.
“Maybe we can help first responders directly,” Lattanzi said. “They have cameras in the arena, and we could use those. We could use computer vision methods to extract 3D data from the camera and use that inform the command center.”
Clearly, there’s a lot of potential still untapped, but there isn’t likely to be a mad rush to laser scan every significant building in every metropolitan area in the United States this year. The Memphis project got a year’s extension to keep exploring possibilities. The Hancock County project still has post-processing work to do before they can really start doing serious testing. The Center for Advancing Human Machine Partnerships is just getting up and running and there are other universities similarly putting together academics from different disciplines to explore how humans can benefit from artificial intelligence gleaned from real-time data.
“But if you’re asking if that’s anywhere close to reality right now, I would say no,” said Lattanzi.